Why Neural Nets are not like the human brain
.. and why you should not claim they are ..
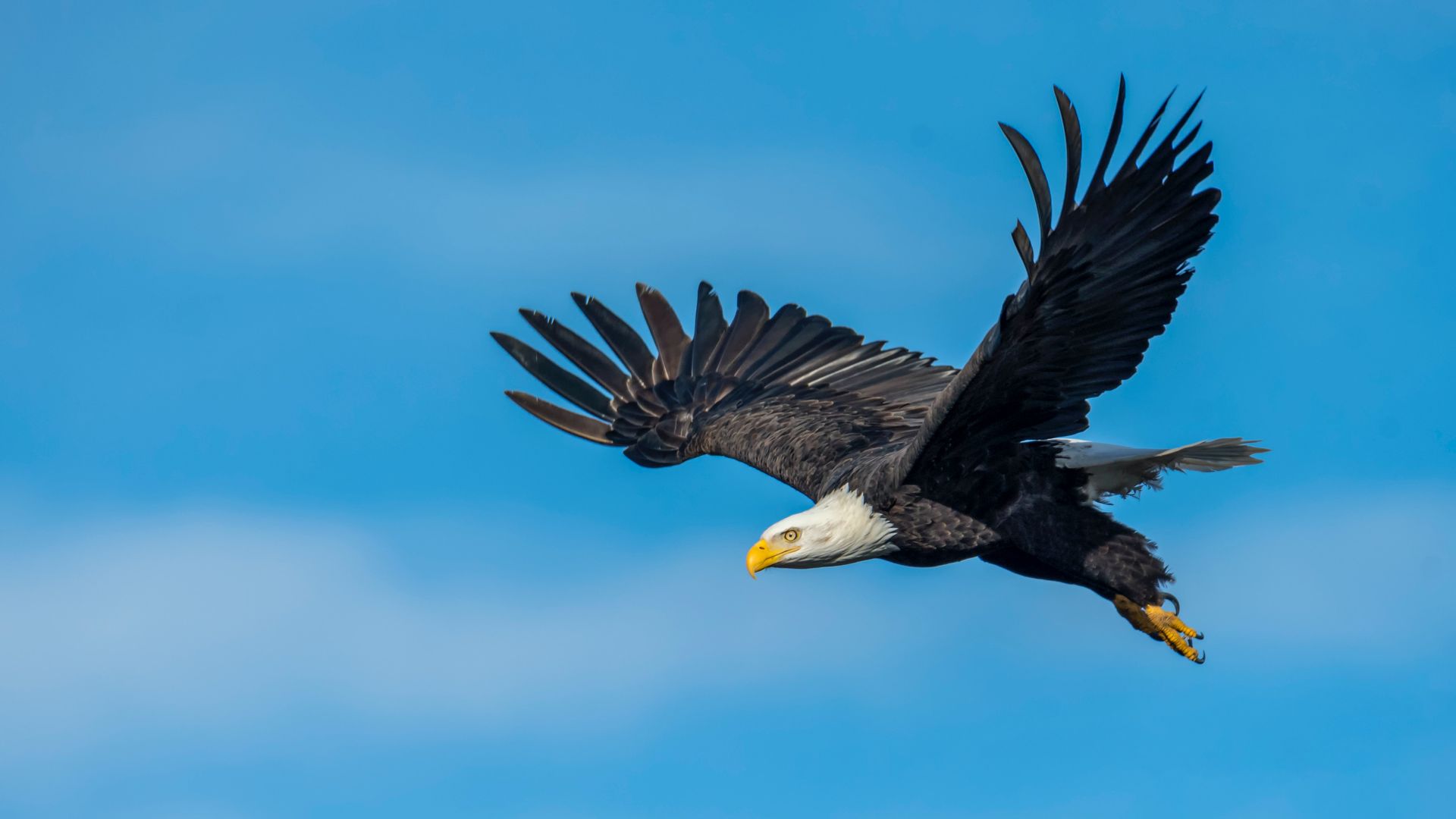
Airplanes are modeled after, and have the same structure, as birds: they both have wings and use air to fly. Airplanes also communicate with each other, just like birds do. Airplanes got better and better in the last 100 years and outperform birds because they can fly quicker; they probably will learn to lay eggs soon and propagate. And, as birds have animal rights, planes should have rights too.
Hopefully you see that I am overextending the analogy. Although some aspects of birds and planes seem the same, that does not mean that one can transfer all features of birds to planes. And even the aspects that are the ‘same’, like wings, are only really the same in name.
At a closer look there are very fundamental differences: birds are biological creatures with wings, feathers, and muscles that enable them to fly by flapping their wings. Birds can take off and land from various surfaces, including tree branches. Birds can glide, soar, and perform acrobatic manoeuvres while using minimal energy. To name a few.
While in the media luckily nobody is stressing the analogy between birds and planes - for obvious reasons- , on a related topic it creates unrealistic expectations, unneeded fears, dangers and futile hope on a daily basis. I am talking about the comparison of AI (and Neural Nets in particular) with the human brain. While (the marketing of) artificial neural networks draw inspiration from the brain's basic structure, they are fundamentally different in terms of architecture, learning mechanisms, energy efficiency, generalization capabilities, the reflection of consciousness and emotions and underlying mechanisms. Neural networks in computers are highly specialized tools designed for specific tasks, whereas the human brain is a versatile and adaptable organ capable of a wide range of cognitive and emotional functions.
To spell it out, some of the key differences are:
Architecture:
- Neural Network Structure: NNs are designed with a specific layered architecture, consisting of input, hidden, and output layers. Each layer consists of interconnected artificial neurons (nodes or units). The architecture is typically feedforward, meaning information flows in one direction from input to output layers. This design is often static and determined by the programmer or designer.
- Human Brain Structure: The human brain is highly complex and interconnected. It doesn't have a simple layered structure like NNs. Instead, it consists of approximately 86 billion neurons, each connected to thousands of other neurons through a vast network of synapses. The brain's structure is dynamic, with connections forming and breaking based on experience and learning.
Learning Process:
- Training in NNs: NNs learn through supervised or unsupervised learning algorithms, where weights and biases of artificial neurons are adjusted during training based on error feedback. Learning in NNs is typically a form of optimization, where the goal is to minimize a predefined objective function
- Learning in the Brain: Learning in the human brain is far more complex. It involves a combination of synaptic plasticity, neurotransmitters, and other biochemical processes. The brain adapts and learns from experiences, continuously modifying the strength of synapses, forming new connections, and pruning existing ones.
Energy Efficiency:
- Neural Network Efficiency: NNs require significantly more computational resources (power, memory, and processing) than the human brain to perform tasks of similar complexity. Training deep neural networks, in particular, can be computationally expensive and energy-intensive.
- Human Brain Efficiency: The brain is highly energy-efficient, using only a fraction of the power required by large-scale NNs to perform cognitive tasks. This efficiency is partially due to the brain's parallel processing capabilities and its ability to selectively activate neurons only when needed.
Generalization vs. Specialization:
- NNs: NNs are often designed for specific tasks or domains and require substantial data and fine-tuning to perform well. They are typically highly specialized and struggle to generalize beyond their training data.
- Human Brain: The human brain is exceptionally good at generalizing from limited data and adapting to a wide range of tasks. Humans can learn and excel in various domains, often with relatively little initial training.
Consciousness and Emotion:
- NNs: NNs lack consciousness and emotional awareness. They are purely computational tools that process data and make predictions or decisions based on mathematical algorithms.
- Human Brain: The human brain can show the reflection of consciousness, self-awareness, and experiencing emotions. These complex phenomena are not understood in their entirety, and are probably not physical by nature.
Processing Speed:
- NN: While modern hardware accelerators like GPUs have greatly improved the processing speed of NNs, they are still fundamentally different from the brain's parallel processing capabilities. NNs typically perform computations sequentially, one layer at
- Human Brain: The human brain is capable of parallel processing and can perform many different types of computations simultaneously. It can process sensory information, control body functions, and engage in complex cognitive tasks all at once.
Underlying mechanisms:
- Human Brain: The human brain's functioning is primarily based on biochemical reactions and the flow of ions across neuronal membranes. Neurons communicate with each other through electro-chemical signals. The release of neurotransmitters, the activation of receptors, and the modulation of ion channels play a crucial role in neural communication. These processes are highly dynamic and complex, involving numerous biological molecules and reactions.
- NNs: NNs do not rely on biochemical reactions but on transistors. They are implemented as mathematical models running on digital hardware (such as CPUs or GPUs). The "neurons" in NNs are simplified mathematical functions that process numerical inputs and produce numerical outputs. These operations involve arithmetic calculations, but they do not involve the complex biochemical processes found in biological neurons.
I am not saying that NN’s can’t do great things, au contraire, they can help solve many great problems that we are facing in this world. But persistently comparing NN's with the human brain has great dangers. Overgeneralizing what NN’s can do (it is so smart, it probably knows better what to do than I do), or attributing emotions or consciousness to it (it is disappointed or wants something, especially if we put the NN in a toy), can lead to placing AI in a position where society delegates the question and actions of how the world should look like to AI systems. And because AI systems are quantitative by nature, they cannot answer qualitative questions. As the Scottish philosopher Hume already said: “you can’t derive an ought from an is”. It is up to us to decide what this great tool, AI, should do.
So, please, do not say that Neural Nets are just like the human brain. They are not.
credits photo: Frank Cone
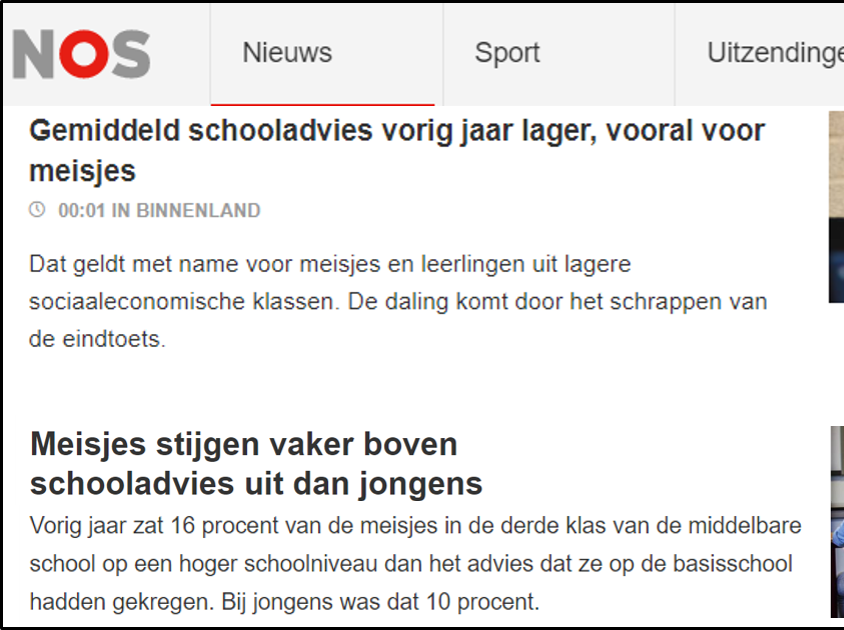
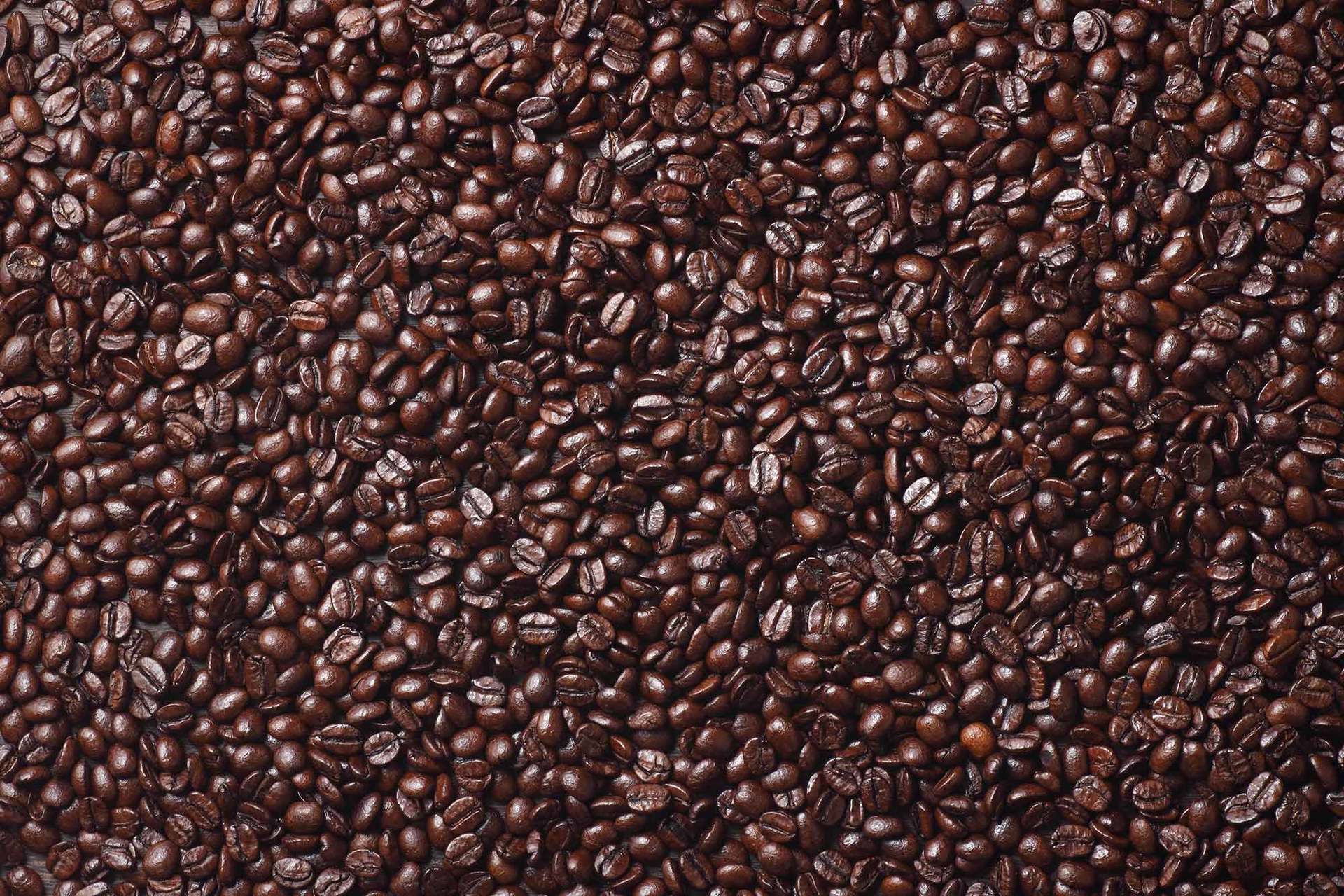
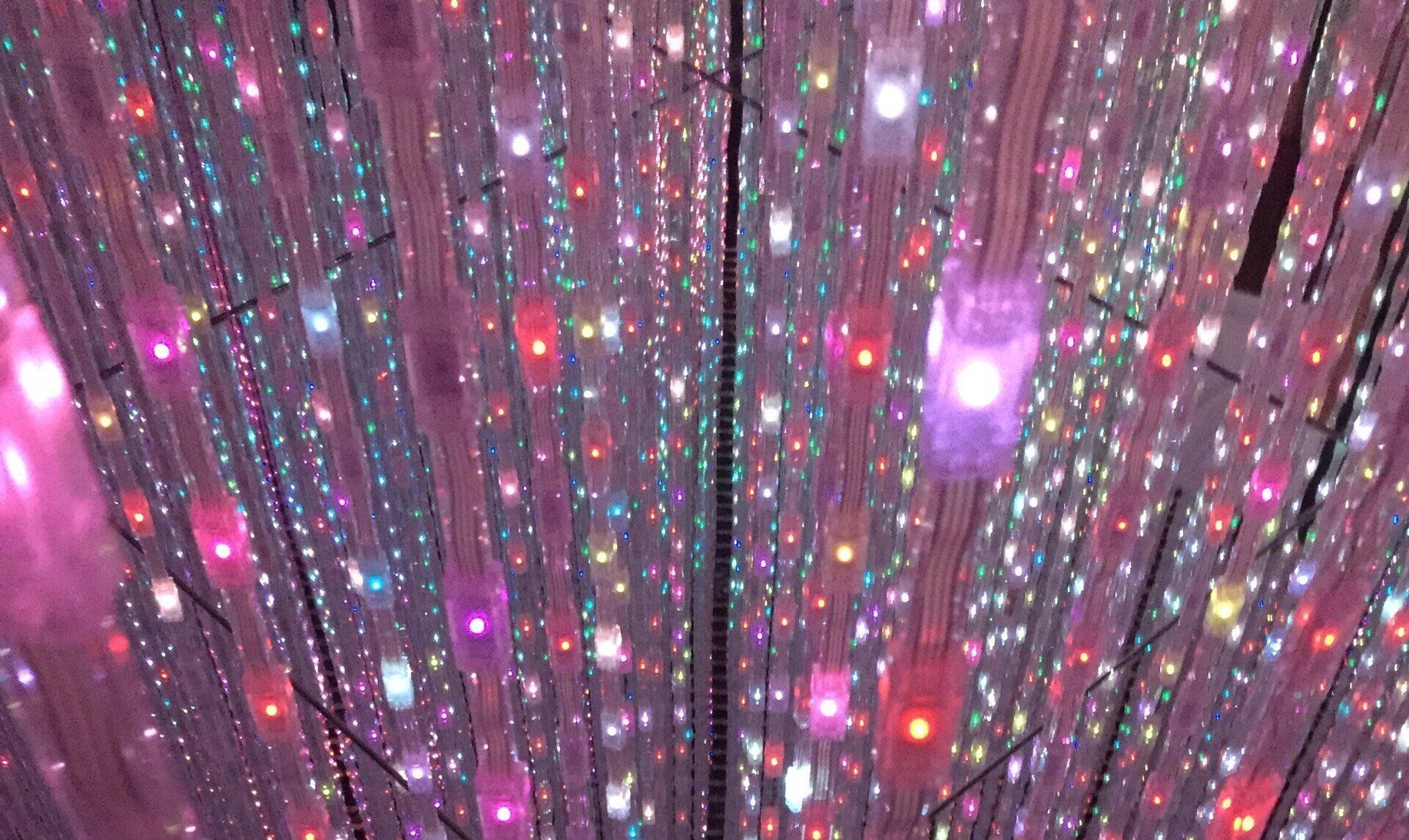
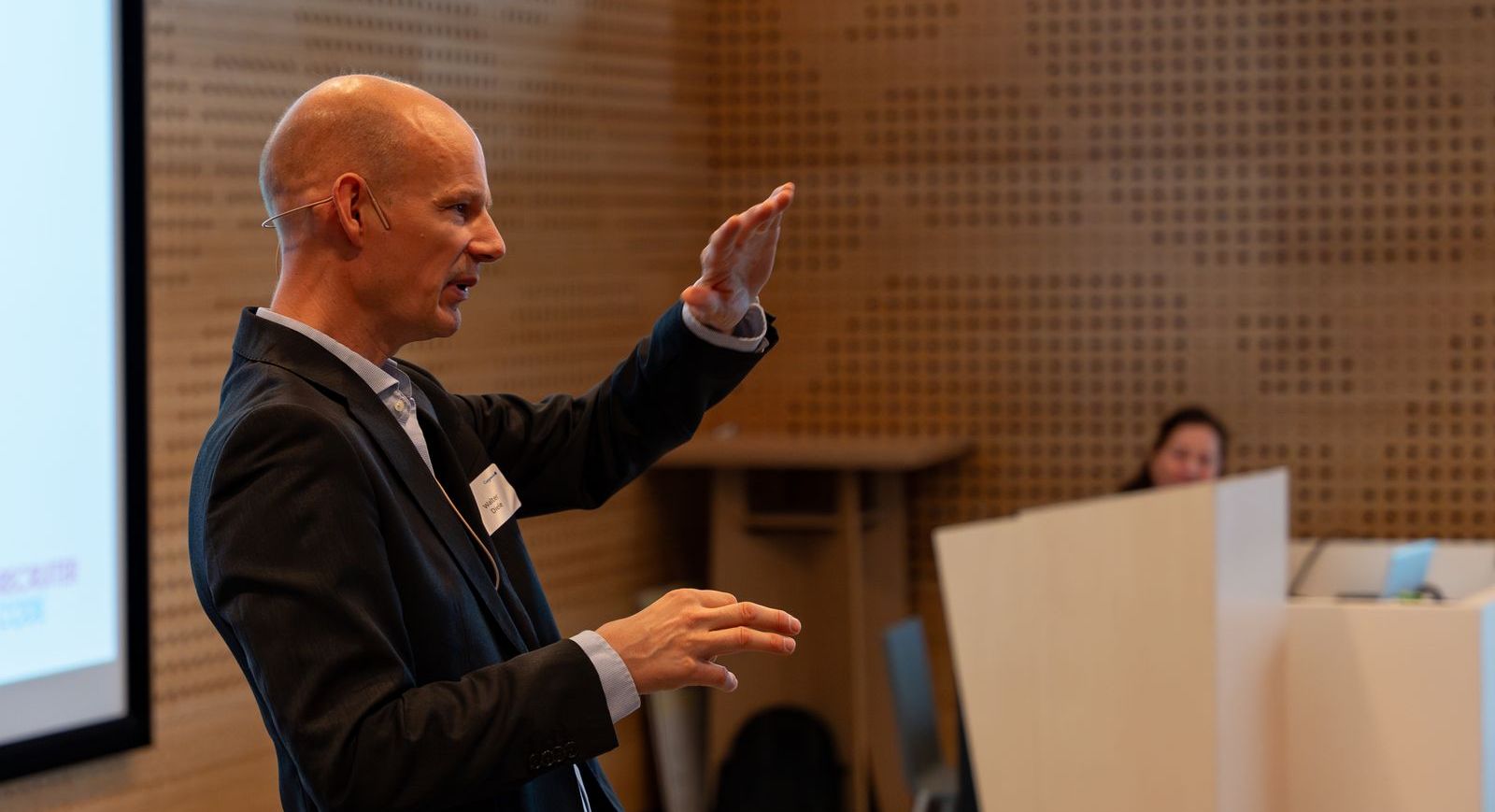
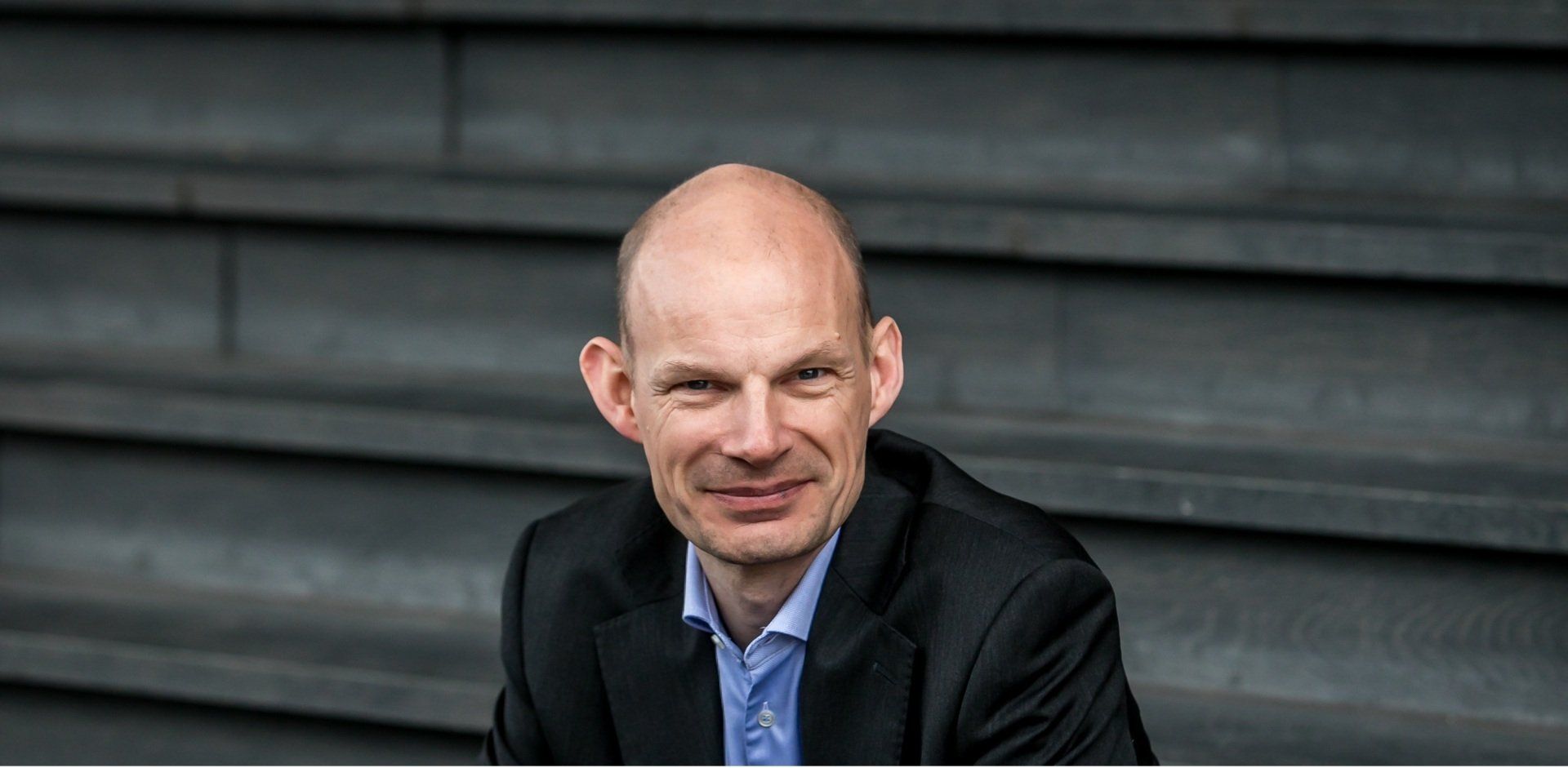
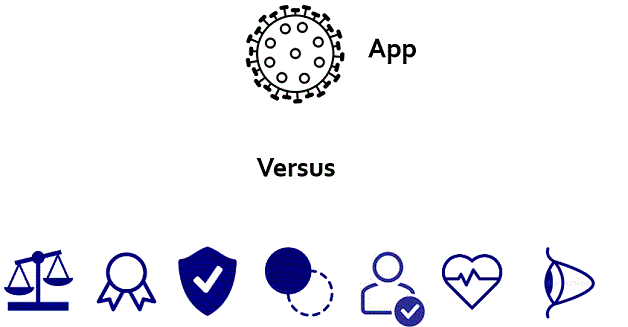
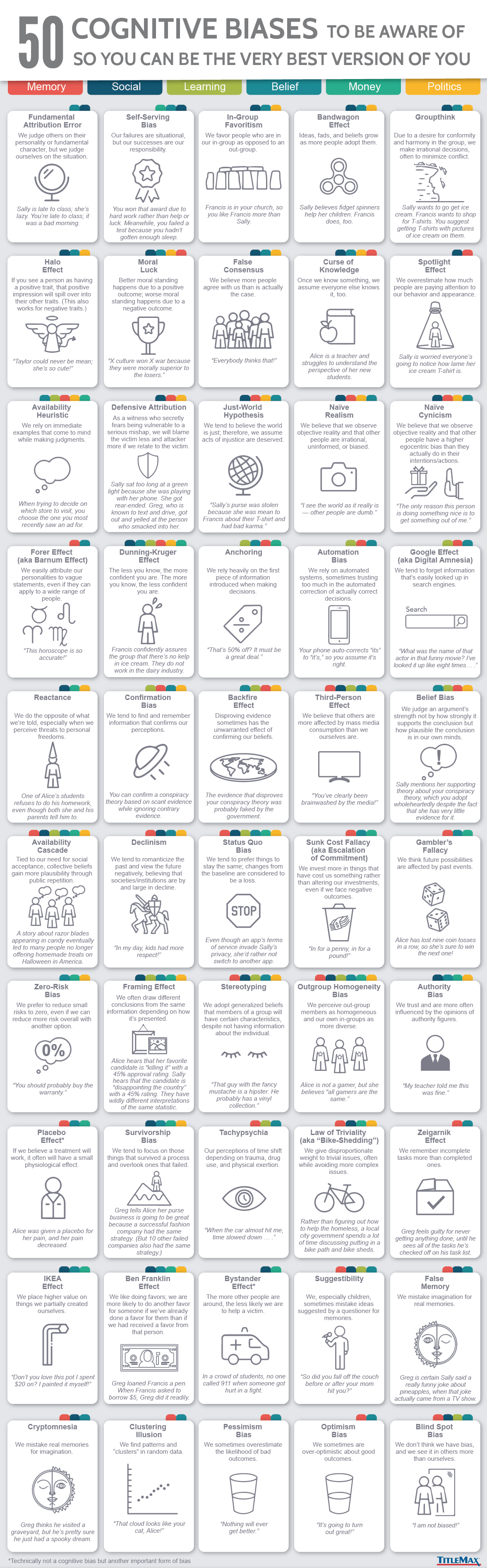